Researchers from UCF’s Center for Research in Computer Vision (CRCV) had a strong showing at one of the top computer vision conferences in the world.
The annual Computer Vision and Pattern Recognition Conference (CVPR), which was held recently in New Orleans, is ranked as the fourth top publication venue among all sciences.
Mubarak Shah, UCF’s CRCV director and a Board of Trustees Chair Professor, organized the event as one of the conference’s general chairs.
Shah, along with three other CRCV faculty members, Department of Computer Science assistant professors Chen Chen and Yogesh Rawat, Department of Electrical and Computer Engineering Professor Nazanin Rahnavard, and 32 doctoral students and alumni, traveled more than 600 miles to experience the conference in person.
Matias Mendieta, one of Chen’s doctoral students, along with doctoral student Taojiannan Yang and researchers from Tulane University and the University of North Carolina at Charlotte, were finalists for the conference’s Best Paper Award.
Their paper ranked 33rd out of 8,161 entries from across the globe.
The paper, “Local Learning Matters: Rethinking Data Heterogeneity in Federated Learning,” is focused on providing insight for improving efficacy of federated learning, which is using collaborative machine learning while keeping new data local and private to a single device. The goal is to facilitate the development of strong machine learning models that can be trained without requiring access to private data.
Mendieta says this has impactful applications in various fields, such as medicine, where data often cannot be shared with other entities.
“Attending the oral presentations and poster sessions was insightful and inspiring,” Mendieta says. “I was grateful for the opportunity to present our work as an oral presentation during the conference.”
Computer science doctoral student Akash Kumar attended and presented his poster at the conference. His work, “End-to-End Semi-Supervised Learning for Video Action Detection,” explores how researchers can approach a problem with fewer labels.
“Video-action detection is a difficult task since it requires a lot of annotated, labeled data, which is extremely expensive,” Kumar says. “My approach investigates how we can achieve the same performance level but with much less labeled data, especially, how we can utilize unlabeled data efficiently.”
In addition to experiencing high quality research and interacting with top researchers, students were able to speak with the numerous companies also in attendance. This was particularly important to Kumar, who plans to join industry after he graduates.
“I got to meet many professors who are experts in their respective research field, interacted with a lot of companies, and got to know what research industries are currently focused on and how they are tackling those problems in the real-world,” Kumar says. “It was nice to talk with senior Ph.D. students who can guide you how to do research and make consistent progress, since they are in the same boat as us. They helped me look at my research problem from a different perspective.”
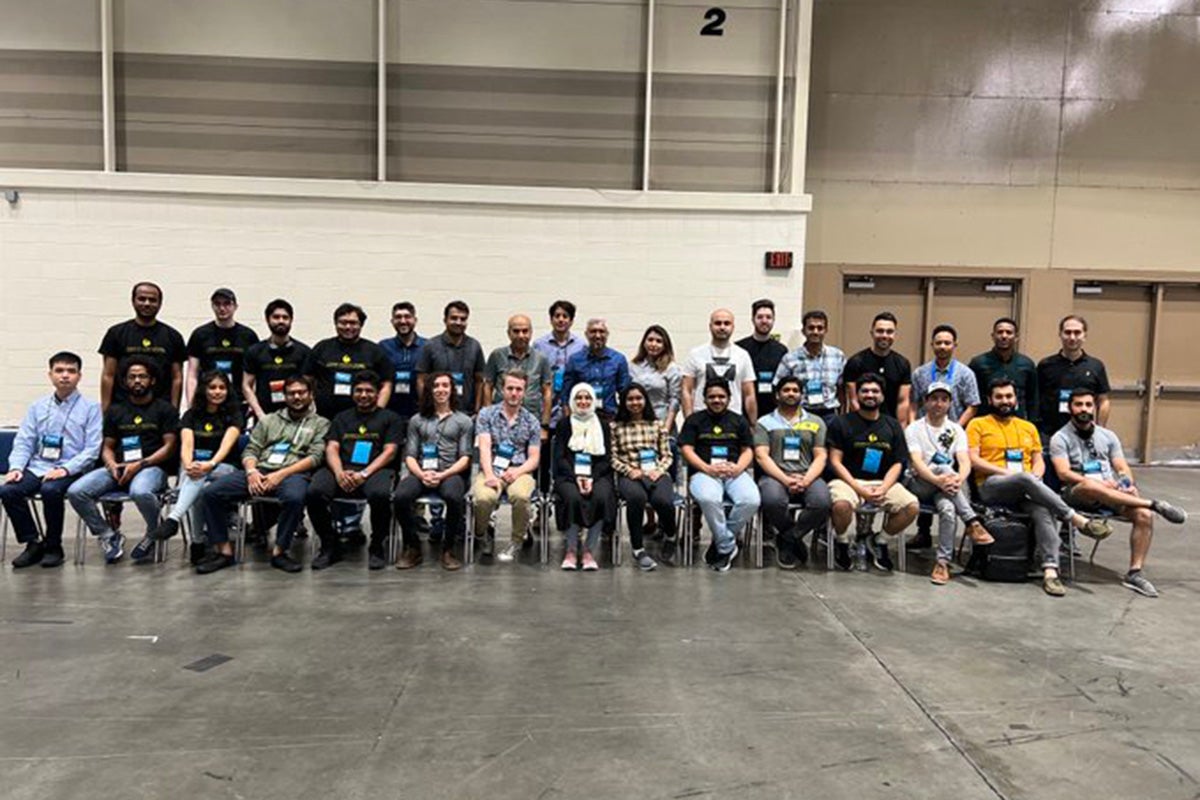
Alongside Shah, who acted as a General Co-Chair for the research conference, assistant professors Chen and Rawat both organized one-day interactive workshops.
Chen’s workshop, “Dynamic Neural Networks Meet Computer Vision,” brought together emerging research in the areas of dynamic deep neural networks optimization, predictive control, dynamic neuro-symbolic reasoning and computer vision in order to discuss open challenges and opportunities ahead.
Chen also was the lead organizer for “First International Workshop on Federated Learning for Computer Vision (FedVision),” a workshop on federated learning and how it can help keep information private.
Rawat organized a workshop titled “Robustness in Sequential Data.” Robustness is an important step toward developing reliable systems that can be deployed in the real world. This workshop encouraged researchers to explore robustness of models against real-world distribution shifts while operating on video and language based sequential data.
He was also a part of organizing the “Tiny Actions Challenge,” a focused task in a series of challenges that aims to recognize small, or tiny, actions in low-resolution videos that are not distinctly visible.
More than 5,500 people attended the conference in-person with another 2,000 joining virtually. It had been on a hiatus for three years thanks to the COVID-19 pandemic.
“We were not sure if we should do this in person or not, but finally decided to go ahead with an in-person conference,” Shah says. “It was a great success.”